How Do You Know Which Antibiotic Is More Effective
Abstruse
The increment in antibiotic resistant bacteria poses a threat to the continued use of antibiotics to treat bacterial infections. The overuse and misuse of antibiotics has been identified as a meaning commuter in the emergence of resistance. Finding optimal handling regimens is therefore disquisitional in ensuring the prolonged effectiveness of these antibiotics. This study uses mathematical modelling to analyse the consequence traditional handling regimens have on the dynamics of a bacterial infection. Using a novel approach, a genetic algorithm, the study and so identifies improved treatment regimens. Using a unmarried antibiotic the genetic algorithm identifies regimens which minimise the amount of antibiotic used while maximising bacterial eradication. Although verbal treatments are highly dependent on parameter values and initial bacterial load, a pregnant common trend is identified throughout the results. A treatment regimen consisting of a loftier initial dose followed by an extended tapering of doses is found to optimise the utilise of antibiotics. This consistently improves the success of eradicating infections, uses less antibiotic than traditional regimens and reduces the time to eradication. The utilise of genetic algorithms to optimise handling regimens enables an extensive search of possible regimens, with previous regimens directing the search into regions of better performance.
Introduction
The discovery of penicillin in 1928 dramatically changed human and animal wellness and well being. Since then, the discovery of additional antibiotics has farther increased survival rates in areas such as surgery and during cancer chemotherapy. However, a lack of new antibiotics and an increment in resistance means these advances are under threat1. Resistance to all antibiotics in clinical employ has now been observed2, with the extensive use and misuse of antibiotics being attributed to the spread of these resistant genes3,iv,5. This has caused considerable contend over the future effectiveness in treating bacterial diseases6,7. As such, the World Health Organization (WHO) has identified antibiotic resistance as one of the major health concerns of the 21st Century.
The credible ease at which antibiotic resistance spreads is due to the ability of leaner to acquire additional genes. Genes can be acquired via either mutations or horizontal gene transfer (HGT). While mutations are undoubtedly a source of resistance, HGT is responsible for increased propagation of resistance through bacterial populations8. If bacteria learn resistant genes in an environment where they are beneficial, HGT volition facilitate the spread of these genes within the population9. Sub-Minimum Inhibitory Concentrations (MIC) of antibiotics and the persistence of high levels of antibiotics within the environs have been linked to the emergence of resistant genes10,xi. Antibody treatments must therefore be effective to minimise the influence of an environment which selects for resistance.
Effective antibody treatment regimens consist primarily of two variables: the dose and the duration of handling. For most antibiotics, the manufacturer identifies a traditional handling regimen which is implemented by doctors and veterinarian surgeons when prescribing these antibiotics. These traditional treatment regimens unremarkably consist of a fixed dose administered for a specified duration. Drug efficiency studies are used to determine the dose and elapsing for these treatment regimens. However, one limitation of this arroyo is that information technology only provides information for the regimen being analysed and offers no indication for other potential regimens. AliAbadi and Lees12 highlighted the importance of rational use of antibiotics and the need to incorporate population pharmacokinetic (PK) and pharmacodynamic (PD) information into dosage scheduling. While traditional treatment regimens may be constructive they may not exist the optimal duration or dose at which to administer antibiotics.
Equally the threat of antibiotic resistance spreads the need to optimise antibiotic dosage regimens becomes essential. Mathematical modelling is increasingly beingness used to investigate optimal treatment regimens for antibody therapy13,14,15,16,17. Even so, these studies either omit pharmacodynamic information, by assuming that the antibiotic induced death rate is abiding; or only analyse a very limited number of alternative handling regimens. With no verification that the elapsing or doses chosen are optimal, these studies look for an 'optimal' solution from a option of sub-optimal treatments. This study therefore aims to accost these assumptions by considering antibiotic induced decease as a part of the concentration of antibiotic present and by using a genetic algorithm (GA) to identify optimal treatment regimens. The use of a GA allows for the automated exploration of the vast space of potential treatment regimens, in guild to locate the most efficient ones. The effectiveness of traditional treatment regimens in eradicating bacterial infections will exist analysed and compared to the alternative treatment regimens identified using the GA. This will be the kickoff study examining the employ of a genetic algorithm to optimise antibiotic treatment regimens.
Model
Deterministic
In keeping with previous studies9,14,18,xix a organization of coupled ordinary differential equations are used to describe the dynamics of a population of susceptible (S) and resistant (R) bacteria. As asexual reproduction requires energy it is causeless that the growth rate of bacteria is express and therefore modelled using the standard logistic growth equation. A cost, a, is associated with conveying the genes which infer resistance to antibioticsxx and results in a reduced growth charge per unit for the resistant strain. Genes tin pass from resistant to previously susceptible bacteria through HGT, β, resulting in the loss of susceptible bacteria and the improver of resistant bacteria. There are iii main mechanisms of HGT: transformation, transduction and conjugation. This report does not distinguish betwixt the differing modes of HGT. Both susceptible and resistant bacteria die at a natural death rate, θ, and through exposure to antibiotics, A i (C).
Antibiotics are added to the system in daily doses. When the concentration of antibiotic C(t) =C(t) +D northward , where
and D is a vector of doses D = (D i, D two, …, D 10). Traditional treatment regimens assume that D 1 =D 2 = … =D 10, nonetheless this report relaxes this constraint. Handling regimens within this report are limited to a maximum of 10 doses but this could be increased indefinitely. The full model can therefore be written every bit equations 1, 2 and 3. A total list of parameters and values tin can be found in Table v (see methods section).
Stochastic
Deterministic modelling contains no randomness and every bit a result produces the same effect each time it is run. Provided the population densities are not besides small, deterministic models produce good approximations of the system dynamics. Even so hosts treated with the same handling regimen volition not all respond in exactly the aforementioned style. The small population size of resistant leaner mean that stochastic events may lead to the emergence or extinction of a resistant strain. A stochastic framework was therefore produced for equations 1, 2 and 3. Simulations are run for 30 days to allow for infection to return if treatment regimens are unsuccessful. Each treatment regimen was run 5000 times with the infection either existence eradicated or nonetheless present at the end of the 30 days. The percentage of runs which resulted in eradication of the infection produced the success charge per unit for each regimen. Due to the ability to simulate the model thousands of times the variability within the results is small. Still, the 95% confidence interval for each treatment was calculated in MATLAB using the Clopper-Pearson exact confidence interval21. This method was chosen due the occurrence of success rates close to 100%. The median time to eradication for all successfully eradicated infections was likewise calculated. (This data was not normally distributed and therefore the median was used instead of the mean).
Results
Numerical simulations were run to analyse the effect unlike treatment regimens have on the population size of bacteria within an infection. The success charge per unit and time to eradication of the infection were analysed. Treatment regimens are obtained from traditional regimens and from solutions derived using a GA. The results presented were performed with an initial resistant population of x% of the total bacterial population. When analysed with an initial resistant population of 1% of the total bacterial population the results follow a similar pattern (run across Supplementary Table S1).
Traditional Treatment Regimens
Using traditional handling strategies of a constant dose administered for 10 days the minimum daily dose required to successfully treat the infection is 23μg/ml (Fig. 1). Nether this regimen the infection is successfully eradicated in 99.8% (95% CI: 99.6, 99.9) of cases (n = 5000 for all simulations). Administering 23μg/ml of antibiotics per 24-hour interval increases the concentration of antibiotic within the system over the ten days, reaching a peak of sixtyμg/ml on day 10 (Fig. 1b).
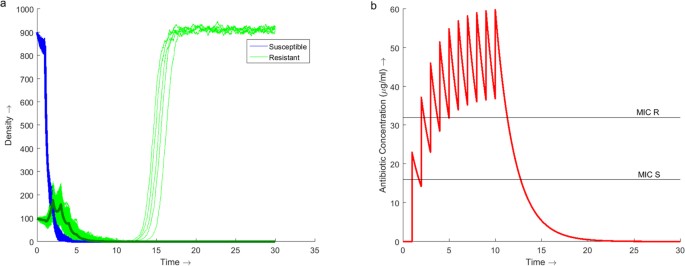
Dynamics of the model over 30 days with antibiotic therapy administered at a daily dose of 23μg/ml for the showtime 10 days.
(a) Stochastic simulations of the population dynamics of both susceptible (blue) and resistant (green) bacteria with the deterministic dynamics (assuming) overlaid. 5000 simulations were run producing a success rate of eradicating the infection of 99.eight% (95% CI: 99.6, 99.9). (b) Simulation of the concentration profile of antibiotic present within the arrangement over the thirty day duration. The MIC lines betoken the concentration of antibiotic required to inhibit the growth of the respective bacterial strain, 16μg/ml for susceptible bacteria and 32μg/ml for resistant bacteria. A maximum antibiotic concentration of sixtyμg/ml is observed on Day ten.
From Fig. 1b it is noted that it takes 3 days before the concentration of antibiotic is maintained above the MIC of the resistant strain. During these beginning 3 days the population of resistant bacteria increases (Fig. 1a). Once above the MIC of the resistant strain the population begins to decrease. If the infection is not eradicated under the traditional treatment regimen so a resistant infection volition emerge.
Until now the study assumed that traditional treatment regimens are administered over x days. This assumption was relaxed and the success rate of eradicating the infection over a shorter duration examined (Table 1). Shorter treatment elapsing results in a decrease in the success rate of eradicating the infection. Handling duration fewer than 8 days experiences a substantial subtract in success rate, to beneath 90%.
The fourth dimension taken to eradicate the bacterial population was also measured. This time was but recorded in the cases where the treatment was successful and the bacterial population completely eradicated. At that place is a small subtract in the time to eradication as the treatment duration decreases from ten days to 7 days. However, this is due to the shorter regimen leading to a lower success rate. The 7 day traditional handling is unable to eradicate infections which persist beyond 8 days due to the antibiotic continuously degrading beyond the last day of treatment. Due to these persistent infections not being eradicated the median time to eradication lowers in comparing to the longer traditional treatment regimens. Equally the treatment length increases above 7 days the success rate besides increases. The median increment in success rate from 8 days to 10 days is iii.4% but requires 18.vii% more than antibiotic to attain this. To maintain a success rate of over 90%, nether a traditional treatment regimen, this infection can be treated past administering a minimum of 184μg/ml of antibiotic over 8 days. This regimen results in a success charge per unit of 96.4% and is used as the baseline to look for improved treatments.
Tailored Treatment Regimens
A genetic algorithm (GA) was used to place constructive dosage vectors, D = (D 1, D 2, …, D 10), which would maximise the success rate of eradicating the infection past minimising the fitness (objective) office (Eq. four).
Minimising the full amount of antibody used, ∑ i D i , exposes the environment to less antibody reducing the likelihood of resistance developing. However, using less antibiotic increases the total bacterial burden on the host over the length of the infection, , where N =Southward +R. The increased bacterial load not simply compromises the health of the host just also offers more opportunity for mutations to arise increasing the take chances of farther resistance developing. A trade-off exists between the full amount of antibiotic used and the total bacterial load over the course of the infection. Weights w 1 and w two allow for more emphasis to exist placed on minimising one term over the other. To ensure a trade-off exists,
and
(However, this written report later considers the case where w i = 0, hence the objective is solely to maximise treatment success.) Due to the difference in the magnitude of the values of each term, correcting factors α ane and α 2 were used to transform the terms between 0 and 1.
Genetic Algorithm with the Deterministic Model
Due to the toxic nature of antibiotics the total antibiotic concentration within the arrangement at whatsoever point in time was constrained to a maximum of 60 μg/ml within the GA. This is in keeping with the maximum concentration from the traditional treatment regimen (although this could be relaxed if needed). The GA was run for varying maximum daily dosages of 60, 50 and 40μg/ml per day. The successful dosage vectors were and so run through a stochastic model to generate a success rate of eradicating the infection.
The dosage vectors from the GA begin with an increased dose which tapers off as the treatment progresses (Table 2). Results from the GA suggests that the elapsing of therapy could be as niggling as 4 days (Tabular array ii, regimens D1 and D3). However, these treatment regimens have a lower success rate, 91.2% (95% CI: 91.0, 92.5) and 92.iii% (95% CI: 91.5, 93.0), than the traditional regimen, 96.4% (95% CI: 95.8, 96.9). For all three maximum daily doses, the longer duration regimens (Table 2, regimens D2, D5 and D8) are more than efficient at treating the infection than the shorter durations with success rates of 94.3% (95% CI: 93.6, 94.9), 94.iv% (95% CI: 93.7, 95.0) and 95% (95% CI: 94.4, 95.half dozen) respectively. The lack of noise inside the deterministic model allows the GA to be very effective in minimising the total antibiotic used. When the shorter dosage vectors from the GA using the deterministic model are analysed using the stochastic model there is too fiddling antibiotic administered over besides short a duration leading to the emergence of resistant bacteria.
The total concentration of antibody in the traditional regimen (Fig. 1b) increases slowly over the eight days. The regimens from the GA start with an initial high dose followed by tapering smaller doses which maintain the total concentration of antibiotic to a higher place the MIC of the resistant bacteria for the majority of the elapsing of handling (Fig. 2). All three regimens D2, D5 and D8 employ less antibiotic in total over a shorter duration than the traditional regimen. Regimen D2 uses thirty% less antibiotic over v days instead of 8. Regimen D5 produces a dosage vector which uses 23% less antibiotic than the traditional regimen and delivers it over vi days instead of 8. The dosage vector from D8 uses 15% less antibiotic and is shorter by 1 mean solar day in elapsing.
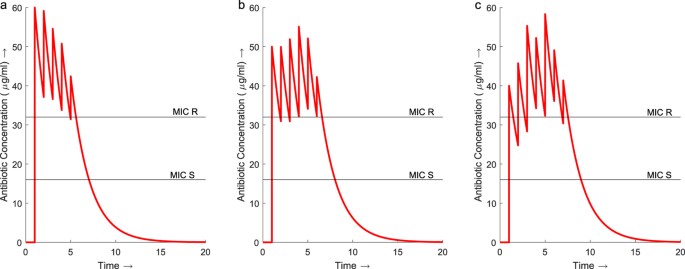
Concentration profiles for regimens D2, D5 and D8 from the dosage vectors identified past the GA with deterministic modelling.
(a) Treatment regimen D2 maintains an antibiotic concentration in a higher place the MIC of the resistant strain throughout the 6 day treatment. The maximum total concentration of antibiotic is 60μg/ml. (b) D5 also maintains a concentration in a higher place the MIC for the resistant leaner throughout the six 24-hour interval handling reaching a maximum total concentration of 54 μg/ml on day 4. (c) The concentration of antibody throughout D8 increases above the MIC of the resistant bacteria initially but drops back beneath for the get-go two days. The concentration is and so maintained above the resistant MIC for the remainder of the treatment, reaching a maximum concentration of 58μg/ml on 24-hour interval 5.
All the regimens identified past the GA see a reduction in the time to eradication for the infection. The median time to eradication for the 8 day traditional handling was vii.13 days (95% CI: 7.04, 7.20). Past distributing the antibiotic in a loftier initial dose with tapering smaller doses the median time to eradication for all the the regimens identified by the GA is between 4 and 5.5 days.
Genetic Algorithm with the Stochastic Model
The GA was run using a stochastic model to maximise the probability of eradication and explore the effectiveness of a longer treatment duration. For the GA using the stochastic model the 2d term, minimising the bacterial load, in F (Eq. 4) was replaced with a term minimising the number of unsuccessful runs out of the 5000. Due to the increased run time, only a few results could be given (Table iii).
The dosage vectors from the stochastic model are noisy due to the randomness in the model. Despite this, the dosage vectors begin to converge to a similar blueprint identified using the GA with the deterministic model. A large initial dose followed by an extended period of tapering lower doses is observed. The median fourth dimension to eradication for the stochastic results are comparable to the deterministic results. Notwithstanding, by using more than antibody over the longer treatment duration the stochastic regimens have a greater success rate. Despite the increase in full antibiotic these dosage vectors utilise between 11 and nineteen% less antibiotic than the traditional regimen with a similar or increased success rate. Dosage regimen S2 has the greatest success rate, 98.four% (95% CI: 97.vii, 98.five), an increase on the traditional 8 day treatment, 96.four% (95% CI: 95.8, 96.nine). The GA was able to place alternative handling regimens using less antibiotic with a success charge per unit of eradication equal to or better than the traditional treatment. The alternative regimens also successfully treat the infection over a shorter duration than the traditional regimen, around 4 to 5 days, vs. 7 to 7.5 days respectively.
If the priority is not to reduce the total antibiotic used, the GA tin can be implemented to maximise the effectiveness of electric current regimens. In this case, how tin can the 184 μg/ml of antibiotics be distributed to maximise the probability of eradication? (i.due east. set w 1 = 0 in Eq. iv) The GA identifies a high initial dose followed by a tapering of doses (Table iii, regimen S4) as the optimal distribution of the antibiotics. This regimen resulted in a success charge per unit of 99.7% (95% CI: 99.5, 99.8) compared to 96.4% (95% CI: 95.8, 96.ix) obtained from the traditional treatment (Table 1). This regimen also eradicates the infection quicker than the traditional regimen with a median time to eradication of 3.94 days (95% CI: iii.89, 3.99) compared to 7.13 days (95% CI: 7.04, 7.19) for the traditional regimen.
Sensitivity Analysis
Due to the difficulty in obtaining exact parameter values for an infection, the effect changes in parameter values have on the success rate of unlike treatment regimens was analysed. Parameter values relating to the virulence of the leaner; replication rate (r), transmission charge per unit (β) and price of resistance (a) were examined. Further sensitivity assay was performed for parameters concerning the effectiveness of the antibioitcs: degradation rate (one thousand), MIC of susceptible (mic Southward ) and resistant bacteria (mic R ) and the shape of the antibody death function (g). Changes in parameters r, a, g and mic R show the greatest change and tin can be found in Figure 3. Other results tin be constitute in Supplementary Effigy S1. Analysis was performed on the traditional eight mean solar day handling regimen (Tabular array 1, regimen T3) and GA generated treatment regimens (Table 3, regimens S2 and S4).
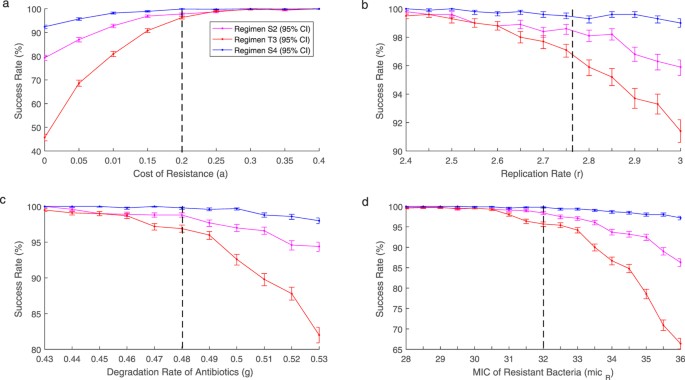
Success rates for regimens S2 (pink), T3 (red) and S4 (blue) at varying values for parameters (a) a, (b) r, (c) g and (d) mic R . Black dashed line shows original parameter values. As parameter values are altered to do good the infection success rates for all three treatment regimens decrease. With the tapered regimens performing better than the traditional regimen. If parameter values are altered to disadvantage the infection the 3 regimens converge to a similar success rate.
As r, grand and mic R decrease, the success rate for all 3 handling regimens converge towards 100%. At these lower parameter values the tapered regimens have no benefit over the traditional regimen. Withal, as r, thou and mic R increase the success rates for all 3 treatments decrease. As the parameter values continue to increase the benefit of the new tapered regimens increase significantly over the traditional regimen. The cost of resistance follows a similar pattern. As a increases the three treatment regimens are every bit every bit effective with all success rates converging to 100%. All the same, when a is decreased the success rates for all three treatments also decrease. Despite the decrease in success rates the tapered regimens obtained from the GA perform ameliorate than the traditional regimen. When there is no price of resistance the success rate of the traditional regimen dropped to beneath fifty% at 45.7% (95% CI: 44.3, 47.i) whereas the tapered regimens remain significantly higher at 79.3% (95% CI: 78.2, 80.four) and 92.4%(95% CI: 91.6, 93.1). Across all the parameter values analysed regimen S4 consistently maintains a success rate in a higher place ninety%. Whereas when the same amount of antibiotic is distributed in a traditional manner the success charge per unit tin drop to beneath l%. Despite regimen S2 using less antibody it besides consistently performs better than the traditional regimen.
While the previous tapered regimens perform well when the parameter values are altered, they are not necessarily the optimal dosage vectors for these new parameter sets. To examine whether the tapered event was a effect of the parameter values chosen the GA was used to generate optimal dosage vectors for the varied parameter values plant in Fig. 3. In every run of the GA, the optimal solution was an initial loftier dose followed past tapering doses. Although the optimal solutions do not change qualitatively, i.e. high dose with tapering, the exact doses practice vary substantially. An case is shown in Table four where the growth charge per unit was varied past x%. Here the same blueprint holds qualitatively only there was variation in the verbal doses. Tapered regimens may exist optimal, withal the verbal doses demand to exist personalised across infections.
Discussion
Current antibiotic treatment regimens consist of a stock-still daily dose administered for a set duration. While these traditional regimens may be easier to administer due to the constant dose, there is picayune evidence that this is the optimal way of administering antibiotics. Despite the continued increase in antibiotic resistance these traditional treatment regimens remain largely unchanged. More research must be defended to ensuring we are using antibiotics in an optimal mode.
This study considered a traditional handling regimen of 23μg/ml of antibody per 24-hour interval for ten days. While this regimen successfully eradicated the infection in 99.8% of cases, the daily dose of antibiotic falls between the MIC of the susceptible and resistant bacteria initially facilitating the emergence of resistance. This is due to the time it takes for the full concentration of antibiotic to increase above the MIC of the resistant strain. While between the two MIC points the susceptible bacteria are eradicated allowing the resistant population to increase with piddling contest. Provided treatment is continued, the concentration of antibody will eventually increase higher up the MIC of the resistant strain.
The GA, using the deterministic and stochastic models, identified that optimal dosage vectors contain an initial high dose followed past tapering lower doses. Initially increasing the concentration of antibody above the MIC of the resistant bacteria eradicates the selective advantage observed in the traditional treatment regimen. Smaller doses of antibiotic are then administered to maintain the concentration above the MIC of the resistant strain. In the example shown the tapered regimens reduce the amount of antibiotic required to successfully care for the infection by as much as 23%. In some regimens produced by the GA, the maximum concentration of antibody within the system was lower than that observed with the traditional treatment regimen (Fig. 2) despite prescribing higher doses. With increased levels of antibiotic selecting for increased resistance, the ability to successfully care for an infection while maintaining a lower total antibiotic concentration over a shorter duration minimises the hazard of higher resistance being selected for.
If traditional handling regimens are adapted to evangelize doses above the MIC of the resistant strain so the initial facilitation of resistant bacteria will disappear. Nevertheless, the full antibiotic concentration within the system will considerably increase. In this scenario increasing the daily dose to above that of the MIC of the resistant strain would increase the full antibiotic concentration to beyond the level determined every bit toxic, 60μg/ml, later three days.
The set duration of treatment is oftentimes subjective with increased length being used as a precaution. Studies accept looked to observe the optimal length of therapy22,23,24 only potential treatment durations are based on empirical bear witness. This study used a mathematical model equally a way to make up one's mind the time to eradication of the infection and therefore the minimum duration of handling required. The 10 day traditional treatment has a median time to eradication of seven.31 days. Additional antibody treatment beyond 8 days resulted in a pocket-size increment in success rate despite a larger increase in total antibiotic required. Whereas treatment length fewer than 8 days (shorter than the fourth dimension to eradication) resulted in a much lower success rate. A traditional treatment regimen of 23μg/ml of antibiotic per mean solar day for eight days was therefore taken equally a baseline handling.
The GA can be used to redistribute the antibody within the traditional regimen to produce a more efficient treatment regimen. The 8 mean solar day traditional handling used 184μg/ml of antibiotics and achieved a success rate of 96.nine% with a time to eradication of seven.thirteen days. The culling treatment regimen identified by the GA applied the 184 μg/ml of antibiotic in a high dose tapered regimen to achieve a success rate of 99.7% with a time to eradication of 3.94 days. This success charge per unit is comparable with the success rate for the 10 solar day traditional treatment but the GA generated regimen uses 20% less antibiotic over fewer days to achieve it. Past redistributing the antibiotic in a high dose, tapered blueprint the fourth dimension to eradication of the infection reduces considerably, allowing shorter treatment regimens to be just as effective.
Studies have shown that shorter treatment regimens tin be effective in treating bacterial infections25,26 with initial loading dose treatments being benign in treating patients in critical care medicine27. Tapered regimens have been constitute to be effective when treating Clostridium difficile 28,29. However, the apply of tapered regimens resulted in sub-optimal functioning in previous studies using optimal control strategies30,31. From the sensitivity analysis it is shown that as the parameters are altered it is possible for the success rate of a tapered regimen to drop significantly. In the example of reducing the cost of resistance the success rate for the tapered regimen dropped to below eighty%. Yet, when the GA is used to place an optimal solution for the new parameter ready information technology produces the same tapered pattern but with different dose values. Generic tapering regimens will not ever be the nigh efficient regimen. The sensitivity in the doses required for a successful tapering regimen indicates that personalisation of individual treatments is required. Such personalisation can be accomplished with the use of a GA. Despite the need for personalisation the tapered regimens consistently performed better than the traditional regimen when the infection was more than virulent or the antibiotic was less effective.
Using a GA to search for an constructive handling regimen immune for a constrained search of all possible dosage vectors. The lack of racket within the deterministic model allows the GA to converge to a specific minimum antibiotic concentration. Notwithstanding, when this is analysed using the stochastic model, random events hateful these treatments are not equally efficient. The stochastic model therefore identifies slightly longer treatments with more antibiotic than the deterministic model, increasing the success rate. The increased computation time of the GA using the stochastic model makes it inefficient. The GA using the deterministic model is much less computationally expensive and produces the aforementioned loading dose but with a shorter tapered duration than the results using the stochastic model. The results from the deterministic model nonetheless have value and provide a suitable starting point from which to base potential future treatment regimens.
The use of the GA suggests that, in order to optimise antibiotic treatment regimens, the idea of constant doses needs to be addressed. Research indicates that the utilize of combination or sequential treatments are more effective in preventing resistance13,15,32. However, these studies apply sub-optimal traditional treatments as comparisons and therefore unmarried antibiotic treatments should not be ruled out. Genetic algorithms provide an efficient manner of identifying and investigating the potential use of culling single, and multiple, antibiotic treatment regimens to prolong the effectiveness of current antibiotics.
Methods
Antibody Expiry Rate
Experimental data33,34 suggests that as the concentration of antibiotic increases the death rate increases until it reaches a saturation betoken. In improver, the concentration of antibiotic naturally decays within a host. The concentration of antibiotic is therefore modelled according to start guild kinetics with an elimination constant m. The one-half-life of the antibody is therefore given by .
To model the relationship between antibiotic concentration and antibiotic induced death rate the extension of the Emax model of antibody handling by Regoes et al.30 was used (Eq. five).
Parameter Values
The parameter values (Table 5) were chosen such that in the absence of antibiotics the resistant strain would not out-compete the susceptible strain. Analytical analysis of the model was performed to identify the conditions which meet this criteria (see Supplementary Equations). This ensures that if resistance invades information technology is due to the treatment regimen and non a issue of the dynamics of the system.
Stochastic Modelling
This study uses the well-established Gillespie algorithm35 to obtain stochastic simulations for the different treatment regimens. By calculating the probability of the individual events occurring, based on rates and parameter values from the deterministic model, the Gillespie algorithm randomly chooses the side by side result to happen and the fourth dimension at which information technology will happen. The population of each leaner is adjusted appropriately and the procedure is repeated. As the events are chosen randomly each simulation will be slightly dissimilar. The success charge per unit for each treatment regimen was obtained by calculating the total number of simulations which resulted in the eradication of both susceptible and resistant bacteria.
Genetic Algorithms
Genetic algorithms were proposed by John Holland in the early on 1970'southward36. They belong to the larger class of evolutionary algorithms, which generate solutions to optimisation problems using techniques inspired past natural evolution, such as inheritance, mutation, selection and crossover37. GAs have previously been used to generate treatment schedules for chemotherapy treatment38,39. Despite being a randomised search GAs are past no means random, instead they apply historical information to directly the search into the region of better operation inside the search space. The GA uses the deterministic model to run simulations using the generated dosage vectors. Values from these simulations are then used to compute the fitness role for that dosage vector. The fettle role of each generated dosage vector are compared with the search space moving towards the vector with the smallest fettle function. (The GA was implemented using MATLAB with a population size of 100, for thousand generations and repeated 50 times with values of 0.01 and 0.99 for Eq. 4, w 1 and w 2, respectively). Varying the weights had no significant effect on the results (run across Supplementary Tabular array S2). Solutions were then run through the Gillespie algorithm to produce a success rate of eradication for each vector.
An alternative approach is to use the stochastic model as part of the fettle part evaluation within the GA. However, the computational fourth dimension increases substantially (in the order of 103) compared to using the deterministic model.
Additional Information
How to cite this article: Paterson, I. One thousand. et al. Optimising Antibiotic Usage to Treat Bacterial Infections. Sci. Rep. 6, 37853; doi: 10.1038/srep37853 (2016).
Publisher's note: Springer Nature remains neutral with regard to jurisdictional claims in published maps and institutional affiliations.
References
-
Teillant, A., Gandra, S., Barter, D., Morgan, D. J. & Laxminarayan, R. Potential burden of antibody resistance on surgery and cancer chemotherapy antibiotic prophylaxis in the Us: A literature review and modelling study. Lancet Infect. Dis. 15, 1429–1437 (2015).
-
Boucher, H. W. et al. Bad bugs, no drugs: no ESKAPE! An update from the Infectious Diseases Society of America. Clin. Infect. Dis. 48, 1–12 (2009).
-
Ferber, D. Superbugs on the Hoof? Scientific discipline 288, 792–794 (2000).
-
Aarestrup, F. M. Veterinary drug usage and antimicrobial resistance in bacteria of brute origin. Basic Clin. Pharmacol. Toxicol. 96, 271–281 (2005).
-
Cabello, F. C. Heavy apply of safe antibiotics in aquaculture: a growing problem for human and animal health and for the surroundings. Environ. Microbiol. viii, 1137–44 (2006).
-
European Committee. Communication from the Committee to the European Parliament and the Council activity plan against the rising threats from antimicrobial resistance 748 (2011).
-
Department of Health & Section for Environment Food and Rural Diplomacy. UK Five Year Antimicrobial Resistance Strategy 2013 to 2018. Dep. Heal. Dep. Environ. Nutrient Rural Aff. 43 (2013).
-
Nakamura, Y., Itoh, T., Matsuda, H. & Gojobori, T. Biased biological functions of horizontally transferred genes in prokaryotic genomes. Nat. Genet. 36, 760–766 (2004).
-
Svara, F. & Rankin, D. J. The evolution of plasmid-carried antibody resistance. BMC Evol. Biol. xi, 130 (2011).
-
Gullberg, East. et al. Pick of resistant bacteria at very low antibody concentrations. PLoS Pathog. 7, 1–nine (2011).
-
Baquero, F., Martínez, J.-L. & Cantón, R. Antibiotics and antibiotic resistance in water environments. Curr. Opin. Biotechnol. 19, 260–five (2008).
-
Aliabadi, F. Southward. & Lees, P. Antibody treatment for animals: Effect on bacterial population and dosage regimen optimisation. Int. J. Antimicrob. Agents 14, 307–313 (2000).
-
Bonhoeffer, S., Lipsitch, Thousand. & Levin, B. R. Evaluating treatment protocols to prevent antibody resistance. Proc Natl Acad Sci USA 94, 12106–xi (1997).
-
Bergstrom, C. T., Lo, G. & Lipsitch, Thou. Ecological theory suggests that antimicrobial cycling will not reduce antimicrobial resistance in hospitals. Proc. Natl. Acad. Sci. USA 101, 13285–13290 (2004).
-
D'Agata, E. M. C., Dupont-Rouzeyrol, M., Magal, P., Olivier, D. & Ruan, S. The bear on of dissimilar antibiotic regimens on the emergence of antimicrobial-resistant bacteria. PLoS 1 iii, e4036 (2008).
-
Geli, P., Laxminarayan, R., Dunne, One thousand. & Smith, D. L. 'One-Size-Fits-All'? Optimizing Treatment Duration for Bacterial Infections. PLoS One 7 (2012).
-
Peña-Miller, R., Fuentes-Hernandez, A., Reding, C., Gudelj, I. & Beardmore, R. Testing the optimality backdrop of a dual antibiotic handling in a 2-locus, two-allele model. J. R. Soc. Interface. 11, 20131035 (2014).
-
Bergstrom, C. T., Lipsitch, M. & Levin, B. R. Natural selection, infectious transfer and the existence atmospheric condition for bacterial plasmids. Genetics 155, 1505–19 (2000).
-
Lili, Fifty. N., Britton, Northward. F. & Feil, E. J. The persistence of parasitic plasmids. Genetics 177, 399–405 (2007).
-
Andersson, D. I. & Hughes, D. Antibiotic resistance and its cost: is information technology possible to reverse resistance? Nat. Rev. Microbiol. 8, 260–71 (2010).
-
Clopper, C. & Pearson, S. The use of confidence or fiducial limits illustrated in the case of the Binomial. Biometrika 26, 404–413 (1934).
-
Lutters, M. & Vogt-Ferrier, North. B. Antibiotic duration for treating uncomplicated, symptomatic lower urinary tract infections in elderly women. Cochrane Database of Systematic Reviews 3, Fine art. No.: CD001535 (2008).
-
Plummer, A., Wildman, Grand. & Gleeson, T. Elapsing of intravenous antibiotic therapy in people with cystic fibrosis. Cochrane Database of Systematic Reviews nine, Fine art. No.: CD006682 (2016).
-
Yuan, Y. et al. Optimum duration of regimens for Helicobacter pylori eradication. Cochrane Database of Systematic Reviews 12, Art. No.: CD008337 (2013).
-
Parry, C. M. et al. Randomized controlled comparison of ofloxacin, azithromycin, and an ofloxacin-azithromycin combination for handling of multidrug-resistant and nalidixic acrid-resistant typhoid fever. Antimicrob. Agents Chemother. 51, 819–825 (2007).
-
Briggs, S., Ellis-Pegler, R., Roberts, South., Thomas, Thou. & Woodhouse, A. Brusk course intravenous benzylpenicillin handling of adults with meningococcal affliction. Intern. Med. J. 34, 383–387 (2004).
-
McKenzie, C. Antibody dosing in critical illness. J. Antimicrob. Chemother. 66, 25–31 (2011).
-
McFarland, L., Surawicz, C. & Elmer, G. Breaking the cycle: strategies for 163 cases of recurrent Clostridium difficile. Am. J. Gastroenterol. 97, S157 (2002).
-
McFarland, 50. V. Alternative treatments for Clostridium difficile disease: What actually works? J. Med. Microbiol. 54, 101–111 (2005).
-
Peña-Miller, R., Lähnemann, D., Schulenburg, H., Ackermann, M. & Beardmore, R. Selecting Against Antibiotic-Resistant Pathogens: Optimal Treatments in the Presence of Commensal Bacteria. Balderdash. Math. Biol. 74, 908–934 (2012).
-
Imran, M. & Smith, H. A Model of optimal dosing of antibiotic treatment in biofilm. Math. Biosci. Eng. 11, 547–571 (2014).
-
Kim, S., Lieberman, T. D. & Kishony, R. Alternating antibiotic treatments constrain evolutionary paths to multidrug resistance. Proc. Natl. Acad. Sci. 111, 14494–14499 (2014).
-
Regoes, R. R. et al. Pharmacodynamic Functions: a Multiparameter Arroyo to the Pattern of Antibody Treatment Regimens Pharmacodynamic Functions: a Multiparameter Approach to the Design of Antibiotic Handling Regimens. Antimicrob. Agents Chemother. 48, 3670–3676 (2004).
-
Liu, Y. Q., Zhang, Y. Z. & Gao, P. J. Novel concentration-killing bend method for estimation of bactericidal potency of antibiotics in an in vitro dynamic model. Antimicrob. Agents Chemother. 48, 3884–3891 (2004).
-
Gillespie, D. T. Exact stochastic simulation of coupled chemical reactions. The Journal of Physical Chemistry 81, 2340–2361 (1977).
-
Holland, J. Adaptation in natural and artificial systems: an introductory assay with applications to biological science, command, and artificial intelligence (University of Michigan Printing, 1975).
-
Eiben, A. & Smith, J. Introduction to Evolutionary Computing. Natural Computing Serial (Springer Berlin Heidelberg, 2015).
-
Ochoa, K., Villasana, M. & Burke, Due east. M. An evolutionary approach to cancer chemotherapy scheduling. Genet. Program. Evolvable Mach. 8, 301–318 (2007).
-
Ochoa, M. & Villasana, Thousand. Population-based optimization of cytostatic/cytotoxic combination cancer chemotherapy. Soft Comput. 17, 913–924 (2013).
Acknowledgements
I.K.P. is supported by an Affect Studentship funded by the University of Stirling and the Middle for Environment, Fisheries and Aquaculture Scientific discipline (Cefas).
Author data
Affiliations
Contributions
I.K.P., A.H., K.O., C.B.A., and N.M.H.T. conceived the report; I.K.P. and Thousand.O. designed the genetic algorithm; I.K.P. and A.H. performed the mathematical analysis; and I.K.P. wrote the paper. All authors reviewed the manuscript.
Ethics declarations
Competing interests
The authors declare no competing financial interests.
Electronic supplementary cloth
Rights and permissions
This work is licensed under a Creative Commons Attribution 4.0 International License. The images or other third party fabric in this commodity are included in the article's Creative Commons license, unless indicated otherwise in the credit line; if the cloth is not included under the Creative Commons license, users will need to obtain permission from the license holder to reproduce the textile. To view a re-create of this license, visit http://creativecommons.org/licenses/by/iv.0/
Reprints and Permissions
Near this article
Cite this commodity
Paterson, I., Hoyle, A., Ochoa, One thousand. et al. Optimising Antibiotic Usage to Treat Bacterial Infections. Sci Rep half-dozen, 37853 (2016). https://doi.org/x.1038/srep37853
-
Received:
-
Accepted:
-
Published:
-
DOI : https://doi.org/10.1038/srep37853
Farther reading
Comments
By submitting a comment you hold to abide by our Terms and Community Guidelines. If you find something abusive or that does not comply with our terms or guidelines please flag information technology as inappropriate.
Source: https://www.nature.com/articles/srep37853
0 Response to "How Do You Know Which Antibiotic Is More Effective"
Postar um comentário